Hive理論基礎
- 2020 年 10 月 10 日
- 筆記
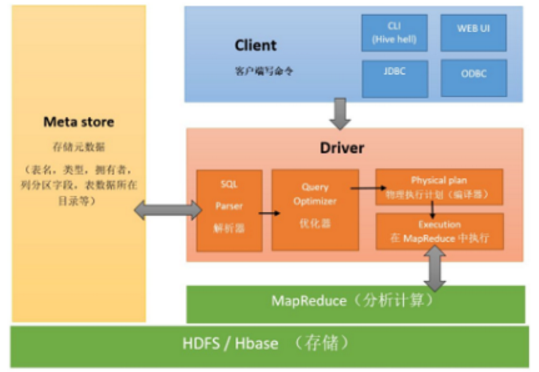
組件:用戶介面,元數據存儲mysql / derby,解釋、編譯、優化、執行器。
與資料庫的區別
- 數據存儲位置不同:Hive存儲在HDFS中,資料庫存儲在塊設備或本地文件
- 數據更新:數倉一般不改寫數據,資料庫增刪改查
- 執行延遲:Hive延遲高, mysql延遲低, 只有大規模數據時Hive並行計算的優點才會體現
- 數據規模:Hive大規模計算,資料庫規模較小
- 內嵌模式:使用的是內嵌的Derby資料庫來存儲元數據,也不需要額外起Metastore服務。
- 本地模式:
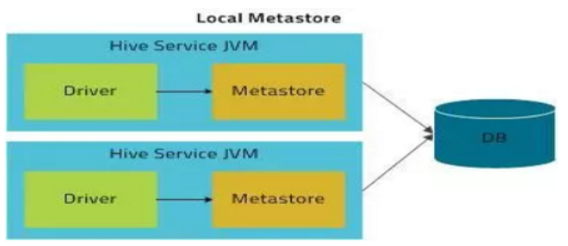
- 遠程模式:
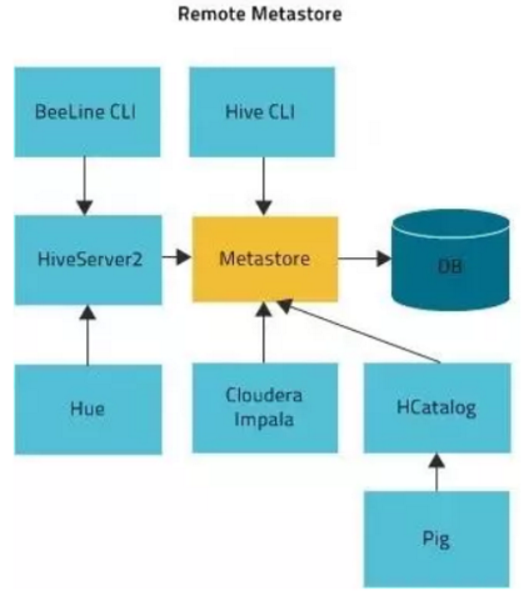
- db(庫):在 hdfs 中表現為 hive.metastore.warehouse.dir 目錄下一個文件夾
- table(內部表):在 hdfs 中表現所屬 db 目錄下一個文件夾,當我們刪除一個內部表時,Hive也會刪除這個表中數據。內部表不適合和其他工具共享數據。
- external table(外部表):數據存放位置可以在 HDFS 任意指定路徑 ,刪除該表並不會刪除掉原始數據,刪除的是表的元數據
- partition(分區):在 hdfs 中表現為 table 目錄下的子目錄
1 create table t_user_part(id int,name string,country string) 2 partitioned by (guojia string) 3 row format delimited fields terminated by ',' ; 4 --注意順序問題 5 --分區的欄位不能是表當中的欄位 6 7 load data local inpath './root/4.txt' 8 into table t_user_part partition (guojia='usa'); 9 10 load data local inpath '/root/5.txt' 11 into table t_user_part partition (guojia='china'); 12 --將數據載入到哪個文件夾中 13 14 --多級分區 15 create table t_order(id int,pid int,price double) 16 partitioned by (year string,month string,day string) 17 row format delimited fields terminated by ',' ; 18 19 load data local inpath '/root/5.txt' 20 into table t_order partition (year='2019',month='09',day='18'); 21 22 load data local inpath '/root/4.txt' 23 into table t_order partition (year='2019',month='09',day='18'); 24 25 ALTER TABLE t_user_part ADD PARTITION (guojia='riben') 26 location '/user/hive/warehouse/hadoop32.db/t_user_part/guojia=riben'; 27 --一次添加一個分區 28 29 ALTER TABLE order ADD 30 PARTITION (year='2018', month='09',day="20") 31 location'/user/hive/warehouse/hadoop32.db/t_order' 32 PARTITION (year='2019', month='09',day="20") 33 location'/user/hive/warehouse/hadoop32.db/t_order'; 34 --一次添加多個分區 35 36 --刪除分區 37 ALTER TABLE t_user_part DROP IF EXISTS PARTITION (guojia=riben); 38 39 --查看分區 40 show partitions table_name; 41 42 show formatted table_name;
- bucket(分桶):在 hdfs 中表現為同一個表目錄下根據 hash 散列之後的多個文件 ,採用對列值哈希,然後除以桶的個數求余的方式決定該條記錄存放在哪個桶當中
1 create table stu_buck(Sno string,Sname string, 2 Sbrithday string, Sex string) 3 clustered by(Sno) 4 into 4 buckets 5 row format delimited fields terminated by '\t'; 6 --clustered by 根據哪個欄位去分桶,這個欄位在表中一定存在 7 --into N buckets 分多少個文件 8 --如果該分桶欄位是string,會根據字元串的hashcode % bucketsNum 9 --如果該分桶欄位是數值類型,數值 % bucketsNum 10 11 create table student(Sno string,Sname string, 12 Sbrithday string, Sex string) 13 row format delimited fields terminated by '\t'; 14 --insert+select 15 insert overwrite table stu_buck select * from student 16 cluster by(Sno); 17 --默認不讓直接使用分桶表
1 --load載入 推薦方式,最常見 (分桶表是不支援load) 2 load data local inpath '/root/hivedata/students.txt' 3 overwrite into table student; 4 --載入本地數據到表對應的路徑下 5 --local表明是本地還是hdfs 6 --overwrite表示覆蓋操作(慎用) 7 8 load data inpath '/stu' into table student_ext; 9 --載入hdfs上的文件到表對應的路徑下(追加) 10 11 --insert + select導入 12 --insert 主要是結合 select 查詢語句使用,將查詢結果插入到表中 13 insert overwrite table tablename1 14 [partition (partcol1=val1,partclo2=val2)] 15 select_statement1 from source_table 16 17 --多重插入 18 from source_table 19 insert overwrite table tablename1 20 [partition (partcol1=val1,partclo2=val2)] 21 select_statement1 22 insert overwrite table tablename2 23 [partition (partcol1=val1,partclo2=val2)] 24 select_statement2.. 25 26 --動態插入 substr(day,1,7) as month,day分區的虛擬欄位 順序需要對應 27 insert overwrite table d_p_t partition (month,day) 28 select ip,substr(day,1,7) as month,day 29 from dynamic_partition_table; 30 31 --指定分隔符(複雜類型的數據表) 32 --表1(包含array欄位類型) 33 --數據: zhangsan beijing,shanghai,tianjin,hangzhou 34 -- wangwu shanghai,chengdu,wuhan,haerbin 35 create table complex_array(name string, 36 work_locations array<string>) 37 row format delimited fields terminated by '\t' 38 collection items terminated by ','; 39 --collection items array集合分隔符 40 41 --表2(包含map欄位類型) 42 create table t_map(id int,name string,hobby map<string,string>) 43 row format delimited 44 fields terminated by ',' 45 collection items terminated by '-' 46 map keys terminated by ':' ; 47 --map keys map中k-v分隔符 48 --數據:1,zhangsan,唱歌:非常喜歡-跳舞:喜歡-游泳:一般般 49 -- 2,lisi,打遊戲:非常喜歡-籃球:不喜歡
顯示自帶的函數的用法:
UDF(User-Defined-Function)普通函數 一進一出
繼承UDF 重載evaluate方法 打成jar包(胖包)上傳到伺服器 將jar包添加到 hive 的 classpath hive>add jar /home/hadoop/udf.jar; 創建臨時函數與開發好的java class關聯 create temporary function tolowercase as 'cn.itcast.hive.UDF_Demo'; (不加temporary就是創建永久函數,需要使用drop手動刪除) 在hql中使用自定義的函數tolowercase ip Select tolowercase(name),age from t_test;
UDAF(User-Defined Aggregation Function)聚合函數 多進一出
UDAF是輸入多個數據行,產生一個數據行
用戶自定義的UDAF必須是繼承了UDAF,且內部包含多個實現了exec的靜態類
UDTF(User-Defined Table-Generating Functions)表生成函數 一進多出
繼承org.apache.hadoop.hive.ql.udf.generic.GenericUDTF, 實現initialize, process, close三個方法。 UDTF首先會調用initialize方法, 此方法返回UDTF的返回行的資訊(返回個數,類型)。 初始化完成後,會調用process方法,真正的處理過程在process函數中, 在process中,每一次forward()調用產生一行; 如果產生多列可以將多個列的值放在一個數組中, 然後將該數組傳入到forward()函數。 最後close()方法調用,對需要清理的方法進行清理 把程式打成jar包 添加jar包:add jar /run/jar/udf_test.jar; 創建臨時函數: CREATE TEMPORARY FUNCTION explode_map AS 'cn.itcast.hive.udtf.ExplodeMap'; 銷毀臨時函數:hive> DROP TEMPORARY FUNCTION add_example; UDTF有兩種使用方法, 一種直接放到select後面(不可以添加其他欄位使用,不可以嵌套調用, 不可以和group by/cluster by/distribute by/sort by一起使用) 一種和lateral view一起使用
1 --select 欄位1, 欄位2, ... 2 --from tabelA lateral view UDTF(xxx) 視圖別名(虛擬表名) as a,b,c 3 --例如 4 select name,subview.* from test_message 5 lateral view explode(location) subview as lc;
1 select col1, 2 max(case col2 when 'c' then col3 else 0 end) as c, 3 max(case col2 when 'd' then col3 else 0 end) as d, 4 max(case col2 when 'e' then col3 else 0 end) as e 5 from row2col 6 group by col1;
1 select collect_set(col3) from row2col_1; 2 --將col3的所有數據放到一個集合中(去重) 3 4 select collect_set(col3) from row2col_1 group by col1,col2; 5 --根據col1,col2進行分組,只有第一列和第二列都相同,認為是同一組 6 7 select col1,col2, collect_set(col3) from row2col_1 8 group by col1,col2; 9 --三列顯示,行轉列 10 11 select col1, col2, 12 concat_ws(',', collect_set(cast(col3 as string))) as col3 13 from row2col_1 14 group by col1, col2; 15 --cast(col3 as string)將第三列變成string類型 16 --因為concat_ws是對於字元串拼接
1 select col1, 'c' as col2, c as col3 from col2row 2 UNION 3 select col1, 'd' as col2, d as col3 from col2row 4 UNION 5 select col1, 'e' as col2, e as col3 from col2row 6 order by col1, col2;
1 select col1, col2, lv.col3 as col3 2 from col2row_2 3 lateral view explode(split(col3, ',')) lv as col3;
--例1 --使用 java.lang.Math 當中的 Max 求兩列當中的最大值 select reflect("java.lang.Math","max",col1,col2) from test_udf; --例2 --準備數據 test_udf2.txt java.lang.Math,min,1,2 java.lang.Math,max,2,3 --執行查詢 select reflect(class_name,method_name,col1,col2) from test_udf2;
- get_json_object(string json_string,string path):第一個參數填寫json對象變數,第二個參數使用$表示json變數表示,每次只能返回一個數據項
1 select get_json_object(t.json,'$.id'), 2 get_json_object(t.json,'$.total_number') 3 from tmp_json_test t;
- json_tuple(string json_string,’屬性1′,’屬性2′)
1 select json_tuple(json,'id','ids','total_number') 2 from tmp_json_test;
1 --從http:www.congiu.net/hive-json-serde/下載jar包 2 add jar 3 /root/hivedata/json-serde-1.3.7-jar-with-dependencies.jar; 4 5 create table tmp_json_array(id string, 6 ids array<string>,total_number int) 7 row format SERDE 'org.openx.data.jsonserde.JsonSerDe' 8 stored as textfile; 9 load data local inpath '/root/hivedata/json_test.txt' 10 overwrite into table tmp_json_array;
1 select cookieid,createtime,pv, 2 sum(pv) over(partition by cookieid order by createtime) as pv1 3 from itcast_t1; 4 --pv1: 分組內從起點到當前行的 pv 累積, 5 --如,11 號的 pv1=10 號的 pv+11 號的 pv, 12 號=10 號+11 號+12 6 7 select cookieid,createtime,pv, 8 sum(pv) over(partition by cookieid) as pv3 9 from itcast_t1; 10 --pv3: 分組內(cookie1)所有的 pv 累加 11 12 select cookieid,createtime,pv, 13 sum(pv) over(partition by cookieid 14 order by createtime 15 rows between 3 preceding and 1 following) as pv5 16 from itcast_t1; 17 --pv5: 分組內當前行+往前 3 行+往後 1 行, 18 --如,14 號=11 號+12 號+13 號+14 號+15 號=5+7+3+2+4=21 19 20 select cookieid,createtime,pv, 21 sum(pv) over(partition by cookieid 22 order by createtime rows between current row and 23 unbounded following) as pv6 24 from itcast_t1; 25 --pv6: 分組內當前行+往後所有行, 26 --如,13 號=13 號+14 號+15 號+16 號=3+2+4+4=13, 27 --14 號=14 號+15 號+16 號=2+4+4=10
- ROW_NUMBER() 從 1 開始,按照順序,生成分組內記錄的序列。 1 2 3 4
- RANK() 生成數據項在分組中的排名,排名相等會在名次中留下空位 。1 2 2 4
- DENSE_RANK()生成數據項在分組中的排名,排名相等在名次中不會留下空位。1 2 2 3
1 SELECT 2 cookieid, 3 createtime, 4 pv, 5 RANK() OVER(PARTITION BY cookieid ORDER BY pv desc) AS rn1, 6 DENSE_RANK() OVER(PARTITION BY cookieid ORDER BY pv desc) AS rn2, 7 ROW_NUMBER() OVER(PARTITION BY cookieid ORDER BY pv DESC) AS rn3 8 FROM itcast_t2 WHERE cookieid = 'cookie1';
1 SELECT * FROM 2 (SELECT 3 cookieid, 4 createtime, 5 pv, 6 NTILE(2) OVER(PARTITION BY cookieid ORDER BY createtime) AS rn1, 7 NTILE(3) OVER(PARTITION BY cookieid ORDER BY createtime) AS rn2, 8 NTILE(4) OVER(ORDER BY createtime) AS rn3 9 FROM itcast_t2 ORDER BY cookieid,createtime) temp 10 WHERE cookieid = 'cookie2' AND rn2 = 2;
Lag(col, n)往前n行
Lead(col, n)往後n行
- 行式存儲
- 列式存儲
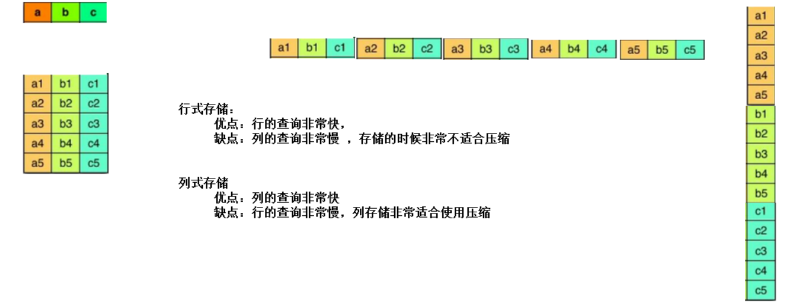
TEXTFILE,行式存儲,但使用這種方式,hive 不會對數據進行切分,從而無法對數據進行並行操作
- indexData:某些列的索引數據。一個輕量級的 index,默認是每隔 1W 行做一個索引。這裡做的索引只是記錄某行的各欄位在 Row Data 中的 offset
- rowData :真正的數據存儲。,先取部分行,然後對這些行按列進行存儲。對每個列進行了編碼,分成多個 Stream 來存儲。
- StripFooter:存放各個stripe 的元數據資訊。每個文件有一個 File Footer,這裡面存的是每個 Stripe 的行數,每個 Column的數據類型資訊等;每個文件的尾部是一個 PostScript,這裡面記錄了整個文件的壓縮類型以及 FileFooter 的長度資訊等。在讀取文件時,會 seek 到文件尾部讀PostScript,從裡面解析到 File Footer 長度,再讀 FileFooter,從裡面解析到各個Stripe 資訊,再讀各個 Stripe,即從後往前讀。
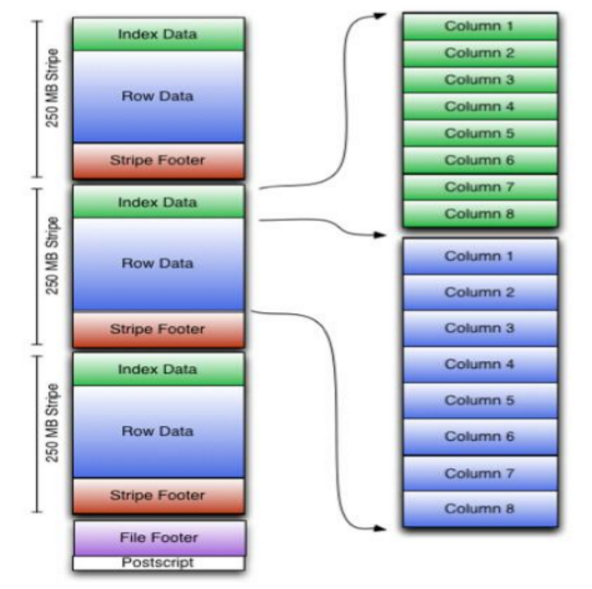
PARQUET,列式存儲,是面向分析型業務的列式存儲格式。Parquet 文件是以二進位方式存儲的,所以是不可以直接讀取的,文件中包括該文件的數據和元數據,因此 Parquet 格式文件是自解析的。 通常情況下,在存儲Parquet數據的時候會按照Block大小設置行組的大小,由於一般情況下每一個 Mapper 任務處理數據的最小單位是一個 Block,這樣可以把每一個行組由一個 Mapper 任務處理,增大任務執行並行度。